Unified approach will be key to telco AI success – UKTIN
Telcos aren't AI native yet. UKTIN's latest telco AI research suggests that data fragmentation and a patch-like approach to applying models are some of the remaining hurdles.
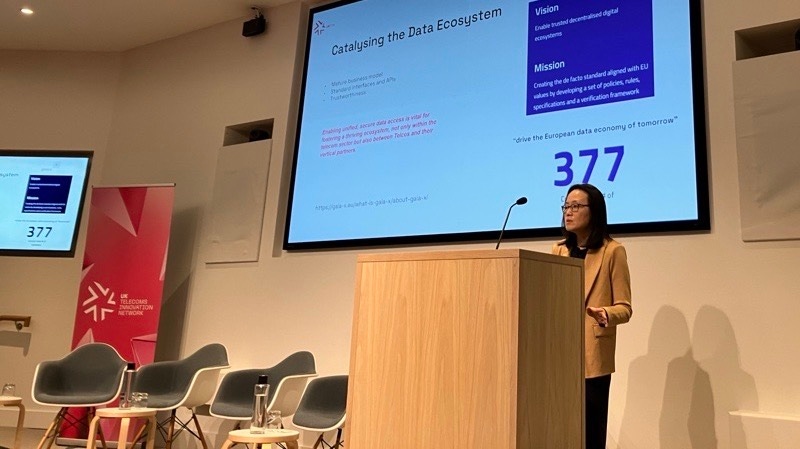
During an event in London last week, the UK Telecoms Innovation Network (UKTIN) unveiled some of its telco AI research findings, due to be published later this month. Yue Wang, Samsung UK's head of advanced network research and chair of the UKTIN expert working group responsible for the research, pointed out that a lot of work is ahead of the industry before it becomes "AI native."
At the moment, Wang noted, AI models are applied separately in patches to individual problems. This leads to a situation where two models may not necessarily work well together, which would lead to yet another patch being applied, increasing complexity.
She said a much better approach would be to have a common framework that would allow AI models to be developed, validated and deployed. This, Wang said, would help overcome initial barriers with data and computational resources. It would also help prevent fragmentation and reduce the cost of integration of different AI solutions at a later date.
Seamless integration
In fact, one of the recommendations in UKTIN's upcoming white paper will be to establish a cohesive testbed for 6G that will facilitate seamless integration and assessment of multivendor interoperability solutions.
At the moment, Wang noted networks are far from being AI native. Once we reach that milestone, however, it will bring its own set of challenges. One of them will be making sure that different AI applications work collaboratively across multiple use cases rather than addressing them separately.
Compatibility will, therefore, be crucial. "Even if I have a perfect AI model that is designed and tested again and again against a particular use case, it doesn't necessarily mean it will work well with other AI products," she said.
The challenge, according to Wang, will be making sure that models trained by different vendors using different data sets in different environments will behave as expected when deployed in the network, given AI is, at the moment, basically a black box.
While a lot of AI training currently happens in the cloud, relying on a centralized server, Wang said that "there is a significant trend where the computational tasks are moving closer to the data source, for example, to the edge and far edge equipment." This helps reduce latency and data passage. Semiconductors can play a significant role, according to Wang, as AI accelerators can reduce costs and power consumption.
She points to privacy and security concerns as a potential challenge arising from working with hyperscalers, despite advantages in the form of avoiding large upfront costs and not having to worry about maintenance. Wang points to the UK government's push to build domestic high performance computing centers, such as in Edinburgh and Bristol, as an alternative.
At the same time, she notes that it is important for these facilities to keep pace with hyperscalers, which tend to update their data centers with the latest GPU.
Data headache
One of the takeaways from the event was that data represents a bigger challenge than computing resources. Apart from GDPR security concerns, the data collected can also be incomplete and difficult to process for various reasons.
Another challenge is data fragmentation, with UKTIN recommending the creation of a data accessibility initiative that would facilitate safe and secure sharing of telco data for the purposes of telco AI R&D and innovation. Wang points to the EU's Gaia-X project as an example that such an initiative could be modeled after.
The issue is that networks generate an abundance of data, with Wang saying they are stored and processed differently in the network, which makes data sharing difficult. While there are standardization activities ongoing to resolve this issue, the fact that several of them are taking place at the same time does not help overcome the challenge.
During a panel session, the University of Edinburgh Associate Professor and Net AI founder and CEO Paul Patras pointed out that the quantity of data available does not necessarily help resolve a problem. Instead, the challenge is to collect the right data needed.
UPDATE: This story has been updated to correct a quote about edge computing and note that computational tasks are moving closer to edge and far edge equipment, not 5G equipment as stated previously. It has also been updated to note that the UK government data center initiative is focusing on domestic high performance computing centers, but not necessarily hyperscalers specifically.
Read more about:
AIAbout the Author(s)
You May Also Like